How to measure ad success and increase ROAS by consolidating data
Vlada Malysheva, Creative Writer @ OWOX
Get in-depth insights
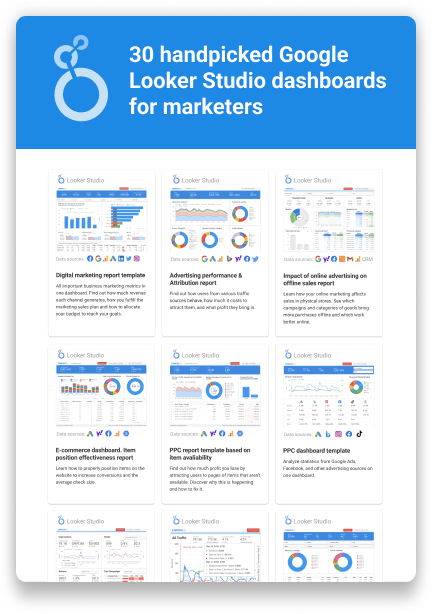
Get in-depth insights
Top 30 Handpicked Google Looker Studio Dashboards for Marketers
Since the more and more businesses transit to online operations and dive into a new, digital world, the more data marketers have to process. Business users are in constant need of high-quality data insights for successful performance and it becomes one of the main challenges to embrace a new analytics formats and structures.
In this case, we describe the solution provided by the OWOX BI team for a leading direct insurance provider that had challenges with evaluating the effect advertising has on purchases.
Goal
As the company sells insurance via the Internet, over the phone, and offline, customers can research products via one channel and purchase them via another. For example, a customer can visit the company’s website, calculate coverage, fill out an online application, and then visit a physical office to make a purchase. In such cases, it’s difficult to evaluate the effect advertising has on purchases.
The main challenge was to optimize ad spending and get a better return on advertising by adopting a functional advertising performance evaluation system. The company needed a way to consolidate data about costs, revenue, and user interactions both online and offline.
Challenge
Data about user interactions with the website, advertising costs, and offline orders is collected in different systems:
- The data from the website is collected in Google Analytics;
- The data about insurance policies purchased offline is stored in the company’s CRM system;
- Non-Adwords cost data isn’t typically collected in Google Analytics. As a result, ROAS, ROI, and share of advertising costs for each ad service are calculated separately.
All this data should be merged to accurately evaluate the contribution of advertising to insurance purchases.
Solution
All the necessary data was collected in Google BigQuery using OWOX BI Pipeline, and implemented the funnel-based, Last Non-Direct Click, and First Click attribution models. Next, the reports were created to compare the campaign performance data obtained via each attribution model.
The data collection and consolidation flowchart is given below:
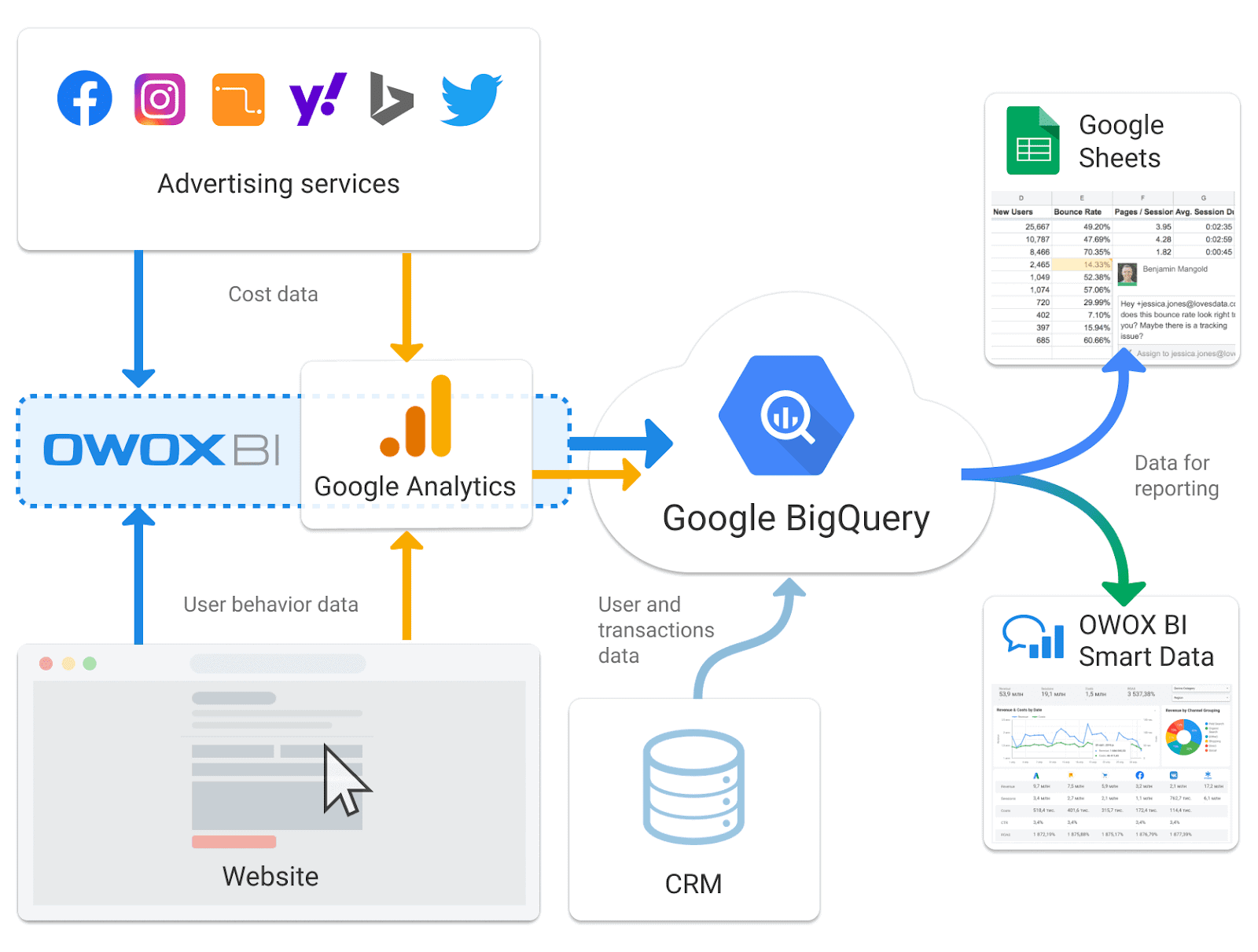
Let’s take a closer look at each step the company took to evaluate advertising campaigns: what data they combined, in what way, and what results were achieved.
Step 1. Collect full user behavior data from the website in Google BigQuery
The company chose Google BigQuery cloud storage for merging data about customers’ on-site activities with data about offline insurance purchases. The service was chosen for its data processing capabilities: terabytes of data can be processed in seconds, petabytes of data — in a couple of minutes. This makes Google BigQuery a perfect service for performing complex analysis and reporting tasks. Another benefit of using Google BigQuery is high security, as the data is automatically encrypted. More information about the benefits of using Google BigQuery can be found on the service’s website.
OWOX BI Pipeline was chosen for data collection for the following reasons:
- Data is collected in near-real time and becomes available within 5 minutes.
- There’s no limit to the number of dimensions and metrics in reporting. OWOX BI Pipeline allows for creating reports of any granularity, using SQL-like syntax to query raw data. In Google Analytics alone, there are certain dimensions and metrics that can’t be combined in one report. There’s a limit to the number of dimensions and metrics in a report: 2 dimensions in a standard report, 5 dimensions in a custom report, and 7 dimensions in a Core v3 request. Moreover, it’s impossible to analyze advertising costs for each individual user. For more information about limits and quotas, see the Google Developers Guide.
- The data imported to Google BigQuery is unsampled regardless of its volume.
More information about the differences between Google BigQuery Export for Google Analytics 360 and OWOX BI Pipeline can be found in our blog post.
Step 2. Collect data from the CRM system in Google BigQuery
The data about insurance purchases is uploaded from the CRM system into Google BigQuery using POST requests.
Step 3. Collect data about advertising expenses in Google BigQuery
- Cost data for AdWords campaigns is automatically collected in Google Analytics due to the native integration between Google Analytics and Google AdWords.
- Cost data for other marketing channels is imported into Google Analytics by OWOX BI Pipeline.
- The data for all channels is imported to Google BigQuery from Google Analytics. This allows for calculating ROAS, ROI, and share of advertising costs, in relation to the purchases, and not only to online applications.
Step 4. Create attribution models
After consolidating the data in Google BigQuery, the marketing team built several attribution models to measure how effective ads are at attracting new customers (the First Click attribution model) and at driving purchases (the Last Non-Direct Click attribution model).
Funnel-based attribution by OWOX BI was chosen as the third, the most objective attribution model. In this model, each channel receives credit depending on its contribution to a customer’s progression through the purchase funnel. Sessions are evaluated based on the probability of the customer’s progression to each next step within the funnel. The higher the probability, the less credit is given to the session which helped a user move on through the step. The lower the probability, the more credit the session gets. Results of calculations within the funnel-based attribution model are available in Google BigQuery and the OWOX BI interface. The report shows the conversion rate (absolute conversion), probability, and value of each step users take on their way to a purchase. Take a look at the example below:
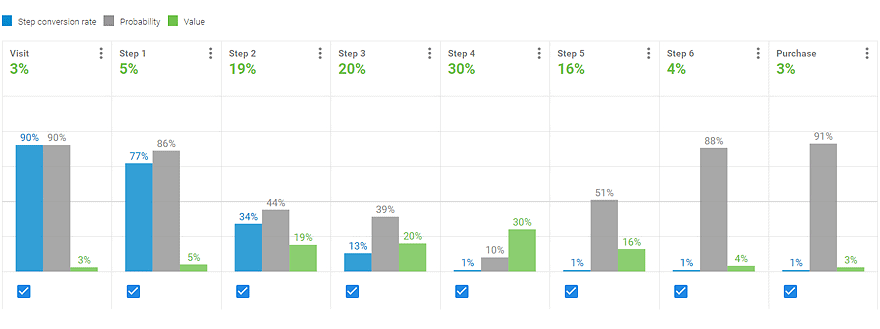
- Step conversion rate is the percentage of sessions in which the given step was viewed. For example, the 34% conversion rate in Step 2 means that 34% of the total number of website visitors navigated to this step.
- Probability is the percentage of sessions in which visitors navigated to the given step from the previous one. In this example Step 4 has a 10% probability, meaning that 10% of visitors navigated to Step 4 from Step 3.
- Value is calculated as follows. First, each step gets a score in inverse proportion to the probability. Then, the score of the step is divided by the total sum of scores within the funnel. The more difficult it is for users to move on through a certain step of the funnel, the higher value it gets.
The OWOX BI Smart Data was used to streamline work with all the obtained data. The tool allows for asking questions to the data in natural English, there’s no need for SQL queries. Question templates make it possible to get answers in a matter of seconds.
By completing the above steps, the company obtained a dashboard that shows both the real value of each channel and the way this value is distributed across funnel steps on different device types.
The graph below shows that the same funnel step can have different values on different devices. The most valuable step on tablets is visiting the website for the first time. Navigating through Step 2 and Step 3 is key for mobile devices. As for sessions on desktop computers and cross-device sessions, the value is distributed more evenly.
What does this translate to? For example, tablet users are less likely to take the first step, i.e., visit the website. This means that ads targeted at tablet devices should be focused on attracting new website visitors.
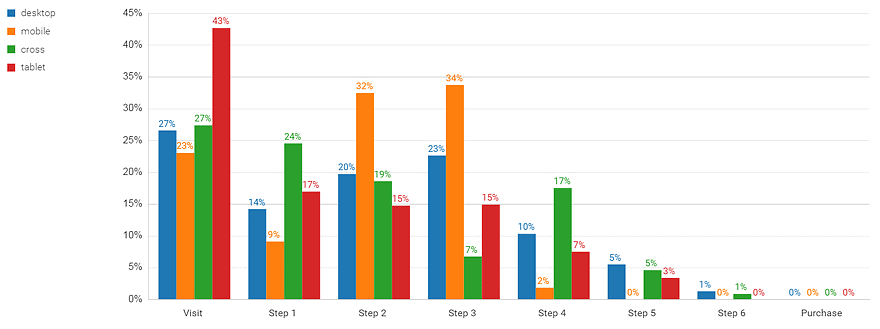
The channel values in the First Click and Last Non-Direct Click models were calculated to identify undervalued and overestimated channels, redistribute the advertising budget more efficiently, and compared them to the results obtained in the funnel-based attribution model.
Neither First Click nor Last Non-Direct Click attribution models can provide an accurate view of campaign performance as Google Analytics doesn’t contain data about insurance policies purchased offline. A user can only go halfway through the online funnel, and then access and purchase the product offline. That’s why the models were implemented and visualized by using Google BigQuery and Google Data Studio, respectively.
By creating a dashboard in Google Data Studio, the company can easily monitor and compare campaign performance for the two most popular attribution models: First Click, in which efforts are focused on the acquisition, and Last Non-Direct Click, in which efforts are directed to purchase. Monthly KPIs are reported in an easy-to-read format.
For example, the report page shown below compares the distribution of revenue and ROAS across different channels. By comparing channel performance in the two different attribution models, one can see that the figures for ROAS and share of the revenue for the channel are almost identical in the two models. This means that these channels are almost equally suitable for both attracting users and pushing them to close the deal.
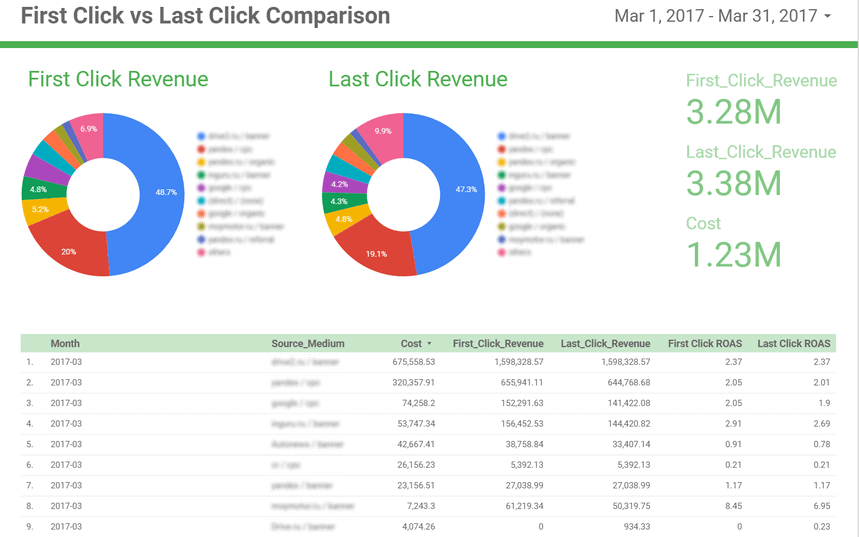
Results
- After fully automating data collection and processing, due to using OWOX BI and Google products, the company team doesn’t have to manually import data. All the data is available in one interface, and marketing specialists can quickly access any data they need.
- The funnel-based attribution model allowed for a more objective and reliable evaluation of marketing channels.
- The team calculated the credit advertising channels would receive in the First Click/Last Click attribution models. By comparing the results to the funnel-based attribution model, the undervalued and overestimated channels were identified, and the advertising budget was reallocated more efficiently.
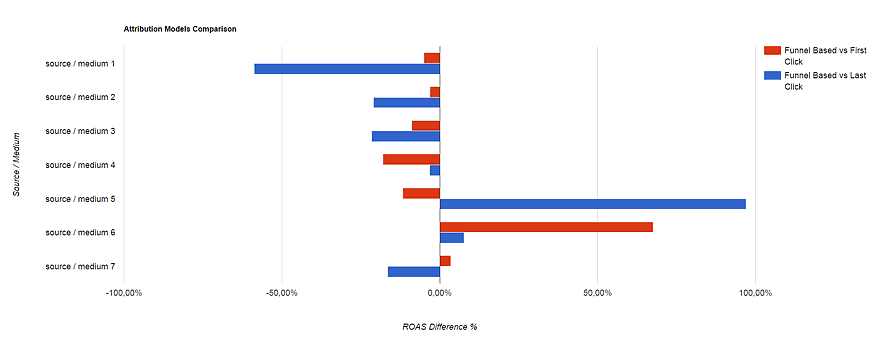
The graph above compares channel values obtained in different attribution models. The contribution of the 5th channel is significantly undervalued in the Last Non-Direct attribution model. According to the funnel-based attribution model, it has a 100% higher ROAS and considerably affects the final conversion. The other channels are mainly overvalued. Based on these findings, the company can reallocate the advertising budget from the overvalued advertising campaigns to the undervalued ones, thus making advertising more efficient without additional expenses.
Get in-depth insights
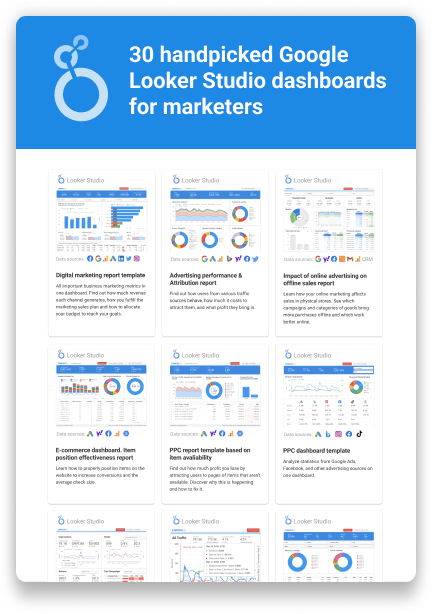
Get in-depth insights
Top 30 Handpicked Google Looker Studio Dashboards for Marketers