How to use customer cohort analysis to measure ad performance
Vlada Malysheva, Creative Writer @ OWOX
Get in-depth insights
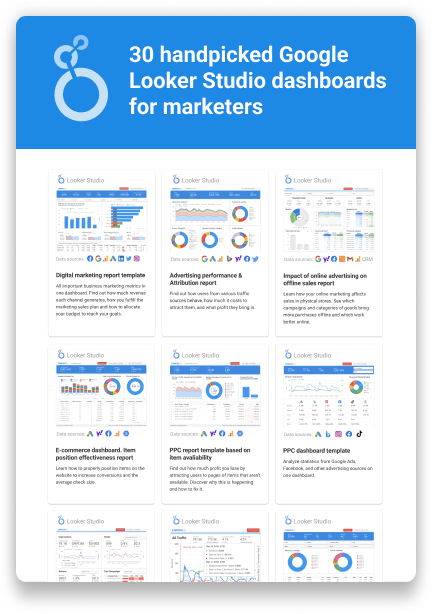
Get in-depth insights
Top 30 Handpicked Google Looker Studio Dashboards for Marketers
Nowadays businesses perform in a digital world, having huge amounts of customer data to gather, save and proceed with scrutiny to detail. It’s not an easy option even for small-size companies, not to mention large enterprises. As business evolves, it also comes to the understanding that it’s possible to advertise more effectively and optimize ad budget.
In this case, we describe the solution provided by the OWOX BI team for a large company supplying an integrated suite of products for business. The suite includes tools for project management, documentation, CRM, telephony, calendars, and more. This client had challenges with spending the advertising budget more efficiently along with conducting a customer cohort analysis.
Goal
As the main challenge for marketing specialists was to reach more customers, everything was done to get as many service registrations as possible. After having gained a lot of active customers in the database, there appeared a necessity to improve the customer lifecycle. The marketing experts wanted to spend their advertising resources only on the target audience ready to buy their product since the customer acquisition costs have increased along with the business competition. Accordingly, most advertising efforts start paying off two months or more after the purchase, as most customers pay for their subscription on a monthly basis.
That’s why, to spend the advertising budget more efficiently, it was decided to conduct a customer cohort analysis. A cohort is a group of users sharing some common characteristics. By grouping users into cohorts based on the time of their first registration, marketers were looking to get more detailed information about what their customers do after they register on the website. Cohort analysis also helps calculate the revenue from each cohort, assess the effectiveness of customer acquisition campaigns, and optimize advertising costs. In addition, comparing behavioral data for the cohorts across different sources, channels, and campaigns helps understand which campaigns work better for customer acquisition and which ones improve retention rate and motivate users to register multiple portals.
Challenge
Since there was a need in providing an effective cohort analysis, all the data should be merged into one system. Another challenge was constantly facing data sampling while working with the free Google Analytics version. The analysts wanted to avoid or at least minimize data sampling without having to export data by day.
Also, a number of specific metrics was required to be included in the cohort analysis report:
- Number of registrations — the number of registered portals.
- MAU — the number of monthly active users.
- CR to MAU — the number of monthly active users as compared to the number of registered users.
- Retention 2nd day — the number of customers that got back on the second day after registering a new portal.
- Share of retention 2nd day — the percentage of customers that re-visit the website on the second day after the registration.
- Paying Users — the number of paying customers.
- CR to Paying — the number of paying users as compared to the number of registered users.
- Revenue SUM — the total revenue generated by a cohort.
- Churn Rate — the percentage of customer churn.
- ROMI — the return on marketing investment, calculated as revenue/costs × 100%.
Solution
The goal was achieved by using the Google BigQuery cloud database in the following way:
- Configuring the import of the customer behavior data and marketing costs data to Google BigQuery using OWOX BI Pipeline.
- Sending user and transaction data to Google BigQuery via the Measurement Protocol.
- Grouping users by cohorts in Google BigQuery and choosing all the necessary metrics.
- Sending the data from Google BigQuery to Google Sheets and creating cohort analysis reports.
The schema below shows the data flow:
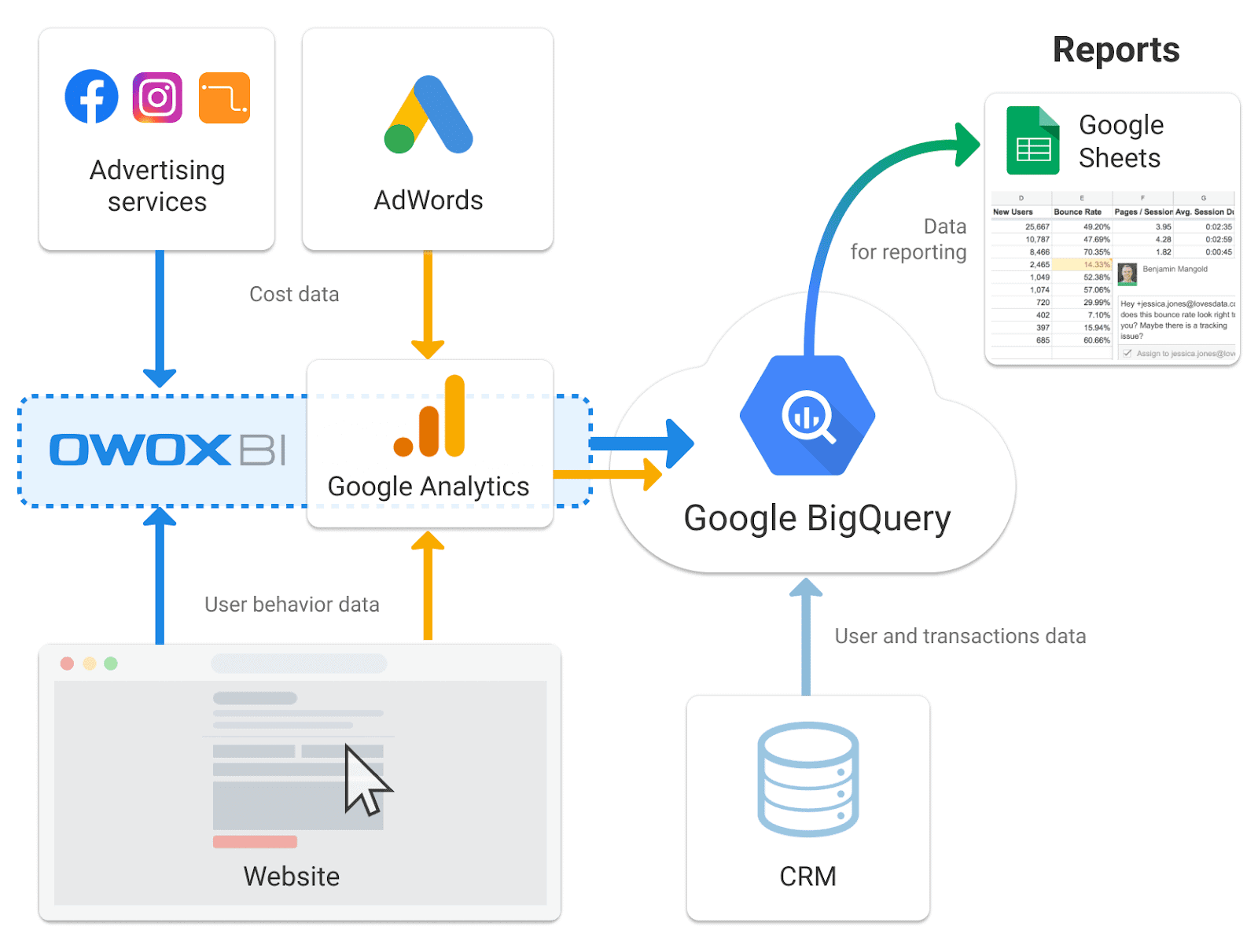
Now let’s take a look at each step in detail.
Step 1. Import data about web user behavior and ad costs to Google BigQuery
Having considered all the alternative platforms, the company chose Google BigQuery for combining the data. The web user behavior data was imported to Google BigQuery using OWOX BI Pipeline. Here are the advantages of this solution:
- No data sampling and limitations on the number of dimensions and metrics in Google Analytics reports. More information about the limitations can be found here.
- Data from the website appears in Google BigQuery within only 5 minutes.
- Revenues and advertising costs can be compared by sessions and users, delivering better insights into the cohort profitability. OWOX BI Pipeline makes it possible to see how much money was spent to acquire each user, by distributing advertising costs over user sessions.
Also with the help of OWOX BI Pipeline, the data from the advertising services is imported first into Google Analytics and then to Google BigQuery. The exception is for AdWords since Google Analytics has native integration with it.
Step 2. Transfer user and transaction data to Google BigQuery
The data about customers is collected in the CRM system. So, the data about the users who re-visit the website on the second day after registering the portal is exported to Google BigQuery. The data about active users and transactions is also exported to Google BigQuery via the Measurement Protocol.
Step 3. Create cohorts and calculate metrics
With all the necessary data collected in Google BigQuery, the cohorts were created and the chosen metrics for each of the cohorts were calculated.
As customers pay for the products monthly, the period of time for analysis was set to a month. Cohorts were created based on the time of the first registration. This means that all users who registered, let’s say in July, belong to the same cohort. With metrics calculated in Google BigQuery, the table has the following structure:
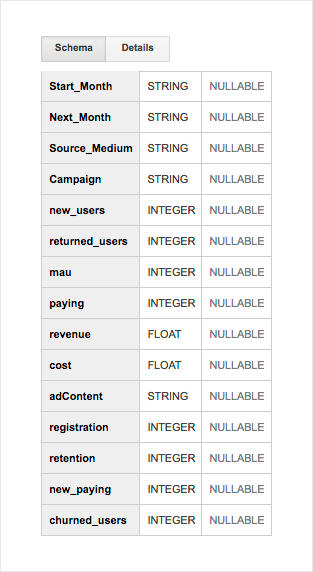
Step 4. Create cohort analysis reports
As the data was exported from Google BigQuery (via the OWOX BI BigQuery Reports add-on), it was visualized in Google Sheets. This data is automatically updated every day and can be filtered by channel, source, campaign, and ad content.
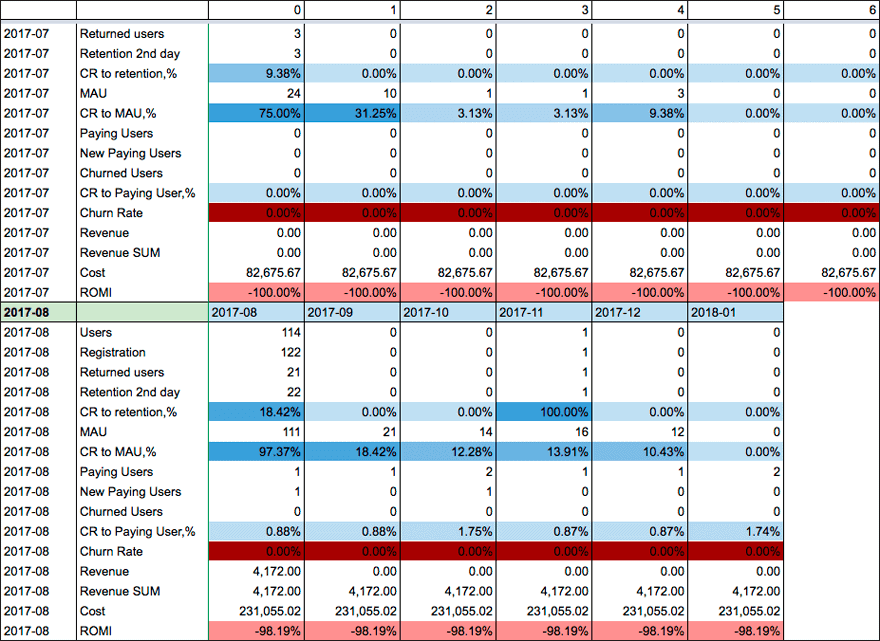
The report demonstrates that the advertising initiatives not only help acquire new customers but also increase customer lifetime value, bringing back already-acquired customers who’re looking to add another portal.
In most cases, the advertising investments start to pay off in three months.
Though the report creation was a difficult task with lots of variable factors, the result was definitely worth it. A set of regular automated reports was created for the marketing department. Before that, marketing specialists had to create such reports manually, double-check everything, and spend a lot of time and effort.
Due to new reports, there also happened some interesting discoveries. For example, it was found that advertising not only works for the customer acquisition but it also contributes to customer retention and helps get more registrations from the same customer.
Results
- The company now has a set of fully automated reports that help measure the performance of advertising channels, sources, and campaigns across customer cohorts on a monthly basis.
- Knowing how well their advertising performs, the marketing specialists can now optimize costs in order to increase the total revenue from each of the cohorts and reduce the churn rate.
- With the help of the cohort analysis, it’s now possible to determine the sources that bring in the most loyal customers. This data can also be used for remarketing.
Get in-depth insights
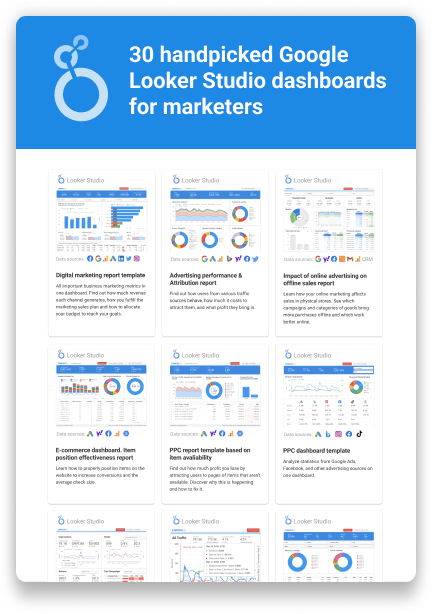
Get in-depth insights
Top 30 Handpicked Google Looker Studio Dashboards for Marketers