How to use advanced analytics to reduce low-quality applications and increase conversions
Vlada Malysheva, Creative Writer @ OWOX
The fast-evolving digital landscape embrace all kinds of business from e-commerce and retail to insurance companies and banks. And as banking services and products become more commoditized, they aim to transform services into an online format along with overall optimizing the business processes. Like any other company coming online, banks also want to maximize their ROI, create new services faster, and gain a competitive edge. For exploring enormous numbers of datasets, banking systems apply advanced analytics.
In this case, we describe the solution provided by the OWOX BI team for one of our bank clients that had challenges with improving the quality of loan forecasting and immediate screening out irrelevant applications.
Task
For the bank, the challenge was in improving the quality of fast customer loan applications on the website. Improved prescoring can reduce the number of failed loan applications and reduce the burden on front-office employees.
The bank used a shortened form with 23 fields, 19 of which are mandatory to fill. In an effort to provide clients with a response that is as close as possible to the decision made in the offline department, the decision was to collect the missing information online (expand the application form to 33 fields with 25 mandatory to fill out). There were fears that a complicated form would reduce the number of questionnaires. At the same time, it was expected that a more informative questionnaire would improve the quality of applications and save time for customers and employees of the bank.
Hypothesis: An expanded loan application form will reduce the rate of conversion to applications, but at the same time will increase the quality of applications.
Research questions:
- How much will conversion to applications decrease?
- Will the quality of loan applications improve?
Solution: running the test
The A/B testing of credit card applications was conducted by launching an experiment with two form options: short and long. In the short form, there were 23 fields, 19 of which were mandatory. The extended form had 33 fields, with 25 being required.
It took 65 days to achieve a minimum detectable conversion change of 1%. The main tracked metric was the rate of conversion to applications since it’s higher than conversion to contracts. Tracking conversion to applications allowed completing the test in a shorter time: the higher the conversion rate, the faster the necessary audience is gathered. To focus on conversion to signing a contract would have meant several months of testing. For the bank, this was too long.
The bank was ready to abandon the expanded questionnaire if the number of credit cards issued decreased. The decrease in conversion to sales was not to exceed the economic benefit of reducing the burden on bank employees.
Since the changes in the form were significant and affected the logic of its work, a server-side replacement mechanism was used to implement the test.
The version of the questionnaire to display to a potential bank customer was determined by a server script and loaded without visible delays for the user.
Google Optimize was used to monitor data and evaluate conversion to applications. Raw data collected in BigQuery using OWOX BI and contract data uploaded from the bank’s CRM system were used to calculate contract conversions. Applications on the website were recorded as Google Analytics e-commerce transactions and thus ended up in BigQuery using OWOX BI Pipeline.
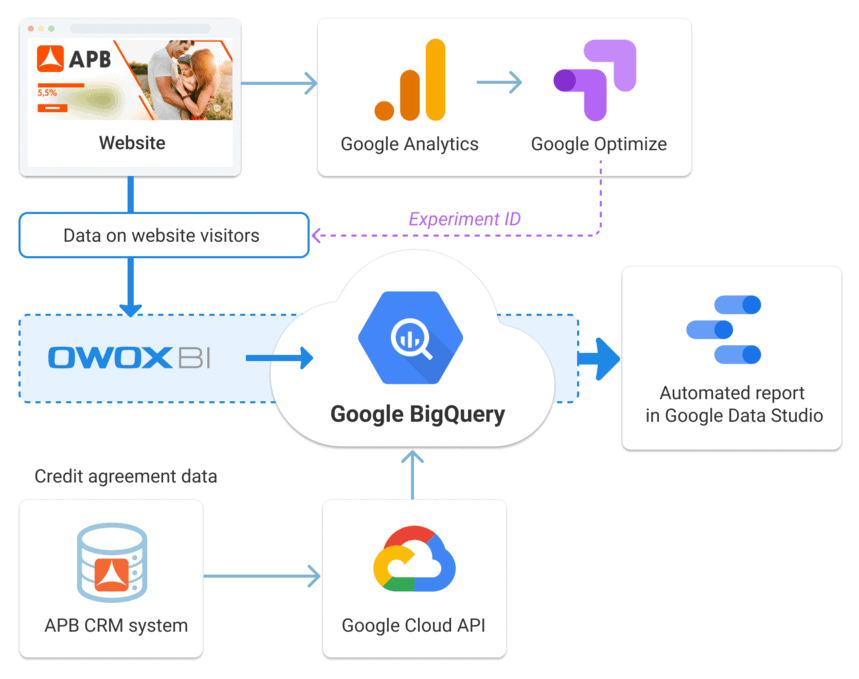
Transaction numbers were sent to the bank’s CRM system. When uploading data from the CRM to Google BigQuery, the transaction number was used as a key to merge data. Based on transaction numbers, all stages of an application could be attributed to the session in which the application was received.
The main advantage of this approach is the absence of a time offset of contracts and applications: the agreement is attributed to the day on which the application was received.
Analysis of results
According to Google Optimize, the conversion rate of the extended application form was expected to decrease by 1%.
Based on test data, it seemed that the test was unsuccessful. Whereas to reach a final conclusion, the team needed to see how experimental applications were converted into contracts. This required additional time, as up to 60 days can pass between the submission of an application and the conclusion of the contract.
The final data showed a noticeable improvement in the quality of applications. Conversion from application to contract increased by 0.52%, while final conversion from visit to contract increased by 0.02%. Customers who completed an extended form were more likely to receive a credit card.
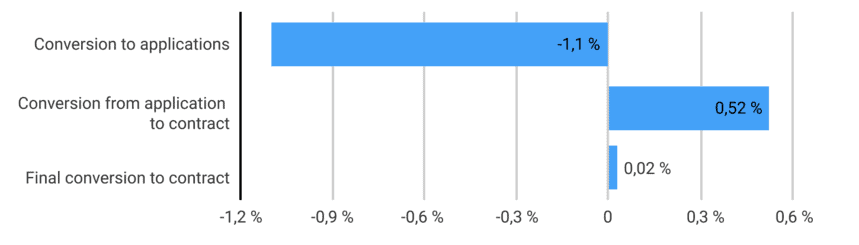
- Conversion to applications — website applications/pilot sessions
- Conversion from application to contract — concluded contracts/applications from the website
- Conversion to contract — concluded contracts/pilot sessions
It’s worth reiterating that the goal was not to increase the conversion rate but to maintain it while introducing a complicated application form. Testing was needed to control the height of the fall.
It turned out that if you evaluate the results relying only on applications, the conversion rate falls. But thanks to advanced analytics, it was possible to track that conversion to contracts not only didn't fall but grew slightly.
Key takeaways
Based on A/B testing conducted, the bank added fields to the application form, deliberately complicating it. It was possible to confirm the bank’s hypothesis that conversion to applications would be reduced but the quality of applications would increase. It was confirmed by collecting raw data using OWOX BI Pipeline and merging that data with contract data in Google BigQuery.
- The new form screened out potentially non-interested customers: conversion to applications decreased by 1.1%.
- The prescoring process improved and the quality of applications improved: conversion from application to contract increased by 0.52%, and the final conversion to contract from lead increased by 0.02%.
- Thanks to the expanded form, the information required for the bank to make a decision is already processed at the online application stage. The load on branch employees has been reduced, and the bank’s costs have been reduced too.
Overall, the change of application form significantly affected the conversion rate. The test showed that when the number of fields increases, mainly low-quality applications are not submitted, which doesn’t affect the final conversion to contract. This means that the bank doesn’t lose anything. Moreover, a long-form increases the final approval rate. It’s important that the number of contracts didn’t decrease when the form was made more complex.