Content
- Challenge #1: Data Retrieval and Storage
- Challenge #2: Data Security Risks
- Challenge #3: Data Integration and Interoperability
- Challenge #4: Data Quality and Accuracy
- Challenge #5: Data Governance and Compliance
- Challenge #6: Data Migration from Legacy Systems to Modern Database
- Challenge #7: Talent and Skills Shortage
- Eliminate Data Transformation Challenges with OWOX BI
7 Biggest Data Transformation Challenges in 2023
Ievgen Krasovytskyi, Head of Marketing @ OWOX
As businesses continue to generate vast amounts of data, managing, securing, and integrating it effectively becomes increasingly complex. This article will outline the seven key challenges that companies are likely to encounter and provide you with practical solutions to help overcome them.
From rapid data growth to data security risks, legacy systems, and talent shortages, we'll dive deeply into each challenge and provide expert tips and guidance to help you manage and optimize your data management strategies.
More to read: The Beginner’s Guide to Data Transformation.
Challenge #1: Data Retrieval and Storage
Data retrieval and storage is a major challenge in data transformation, especially with the rapid growth of data. Here are some solutions for managing rapid data growth:
Design for growth: Organizations must design their data storage systems to accommodate growth. They should plan for at least 10x growth and be prepared to rewrite before 100x growth.
Use efficient retrieval methods: Organizations must use efficient retrieval methods to retrieve data from large-scale data size environments. This can be achieved by using intelligent retrieval methods such as SimHash and multi-attribute decision-making.
Effective data processing: Effective data processing is critical for managing rapid data growth. Organizations must capture data effectively, clean it efficiently, and process it accurately to ensure that their data is accurate and consistent.
Storage choice:Organizations must choose the right storage solution for their data. When choosing a storage solution, they must consider factors such as data volume, data type, and data access requirements.
Challenge #2: Data Security Risks
Data security is a major concern for organizations in 2023. With the increasing amount of data being generated, there is a higher risk of data breaches and cyber-attacks. Organizations must ensure their data is secure by implementing robust security measures such as encryption, access controls, and data masking. They must also comply with data privacy regulations such as GDPR and CCPA.
Risk | Description |
Malware and Viruses | Malicious software that can infect a system and cause damage or extract data. |
Phishing Attacks | Fraudulent attempts to obtain sensitive information (such as login credentials) through deceptive emails or websites. |
Ransomware | Malware that encrypts a system's data and demands payment for the decryption key. |
Insider Threats | Unauthorized access to or misuse of sensitive data by employees or contractors. |
Top 3 tips for mitigating data security risks
Here are the top 3 tips for mitigating data security risks:
Store sensitive data securely: Removable storage devices, including thumb drives and CDs, should be kept with paper files in a secure area such as a locked cabinet, drawer, or safe. Restrict access to these areas to only those who need the information. Organizations must also encrypt their data and create backups to ensure that their data is secure and can be recovered in case of a breach.
Implement firewalls and antivirus software: Firewalls and antivirus software are essential tools for protecting against cyber attacks. They help prevent unauthorized access to IT systems and detect and remove malware and viruses. Organizations must also keep their software updated and use multi-factor authentication to protect against data theft and online attacks.
Conduct regular employee training: Employees are often the weakest link in data security. Organizations must train their employees on data security best practices, such as creating strong passwords, identifying phishing attacks, and reporting suspicious activity. They must also conduct background checks on employees to ensure that they are trustworthy and do not pose a threat to the organization's data security.
By following these tips, organizations can significantly reduce the risk of data security breaches and protect their sensitive data from cyber-attacks.
Uncover in-depth insights
The Beginner's Guide to Data Transformation
Download nowBonus for readers
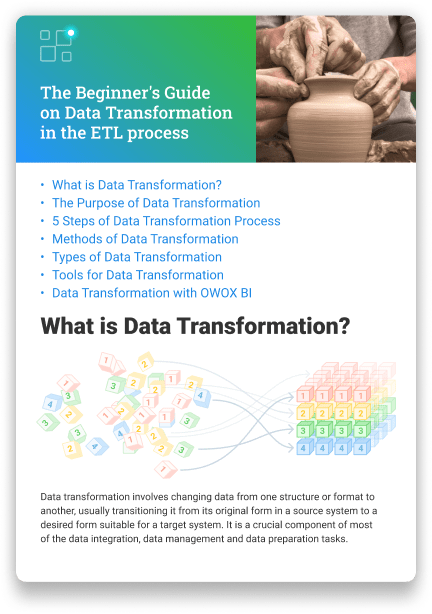
Challenge #3: Data Integration and Interoperability
Data integration and interoperability are critical components of data transformation. However, organizations need help with integrating their data. Two common solutions to address these challenges are breaking down data silos and simplifying data integration with ETL.
Breaking Down Data Silos
Data silos are isolated data repositories that are not integrated with other systems. They can lead to data inconsistencies and inaccuracies, making it difficult for organizations to gain a comprehensive view of their data.
To address this issue, organizations must break down data silos by integrating their data into a single, unified system. This can be achieved by using data virtualization, data warehousing, and data governance.
We’ve prepared the list of 11 best data transformation tools.
Simplify Data Integration with ETL
ETL (Extract, Transform, Load) is a process used to extract data from various sources, transform it into a consistent format, and load it into a target system. ETL tools simplify data integration by automating the process of extracting, transforming, and loading data. They also provide data quality checks and error handling, ensuring the data is accurate and consistent.
Challenge #4: Data Quality and Accuracy
Data quality and accuracy are critical for making informed business decisions and achieving desired outcomes. However, maintaining high data quality can be challenging due to data consistency, errors, and duplication in raw data.
It is essential to establish data governance policies, such as data standards, validation, and cleansing, to improve data quality. Organizations can also leverage data quality tools and technologies that automate data profiling, monitoring, and cleansing processes.
Data Quality Issues
Common Data Quality Issues | Possible Solutions |
Missing or Incomplete Data | Data standardization, validation rules, and mandatory fields |
Inconsistent Data | Establish data standards and enforce data validation rules |
Duplicate Data | Data de-duplication and consolidation |
3 Tips for Eliminating Data Quality Issues
Here are three tips for eliminating data quality issues:
Establish Data Quality Rules: Establishing data quality rules is critical for ensuring that data is accurate, complete, and consistent. Organizations must establish and implement data quality rules across their data transformation processes.
Conduct Regular Data Audits: Regular data audits are essential for identifying data quality issues and ensuring that data is accurate and up-to-date. Data audits can be performed using data quality tools and techniques such as data profiling, cleansing, and validation.
Implement Data Governance: Implementing data governance is critical for ensuring that data is managed effectively and efficiently. It includes data quality, data security, and data privacy policies. Organizations must implement data governance to ensure their data is accurate, consistent, and secure.
Challenge #5: Data Governance and Compliance
Data governance and compliance are critical components of data transformation. Organizations must ensure their data is managed effectively and efficiently while complying with data privacy regulations. Privacy regulations and managing user and data privacy are two common solutions to address these challenges.
Privacy Regulations
Privacy regulations such as GDPR and CCPA require organizations to protect their customers' personal data and ensure it is not misused or mishandled. Organizations must comply with these regulations by implementing robust data security measures, obtaining customer consent, and providing transparency about how their data is being used.
Managing User and Data Privacy
Organizations must manage user and data privacy by implementing data privacy policies, providing training to employees, and conducting regular audits to ensure that their data is secure and compliant with data privacy regulations. They must also ensure that their data is anonymized and encrypted to protect against data breaches and cyber-attacks.
Challenge #6: Data Migration from Legacy Systems to Modern Database
Data migration from legacy systems to modern databases is a common challenge in data transformation. Legacy systems often use outdated technologies and formats, making it difficult to integrate them with modern databases. To address this issue, organizations must develop a data migration strategy that includes data profiling, data cleansing, and data validation. They must also use ETL tools to extract data from legacy systems and load it into modern databases.
Challenge #7: Talent and Skills Shortage
Talent and skills shortage is a major challenge in data transformation. Organizations require skilled professionals with SQL knowledge or Python expertise to manage and transform their data. To address this issue, organizations must invest in training and hiring skilled professionals. They must also provide opportunities for their employees to learn new skills and technologies to keep up with the rapidly changing data landscape.
Eliminate Data Transformation Challenges with OWOX BI
In today's data-driven world, navigating the complexities of data transformation can be daunting. However, with OWOX BI, these challenges become a thing of the past.
Embrace a seamless, efficient, and accurate data transformation experience that empowers your business to make informed decisions.
Don't let data hurdles hold you back.
FAQ
-
Why is data quality important?
Data quality is crucial because high-quality data leads to more accurate analyses, better decision-making, and improved operational efficiency. Poor data quality can result in incorrect insights, misguided strategies, and potential financial losses. Ensuring data is accurate, consistent, timely, and relevant is essential for any organization that relies on data-driven decisions. -
How can data security risks be mitigated?
Data security risks can be mitigated by: Implementing robust encryption: Encrypting data both at rest and in transit ensures that even if data is intercepted, it remains unreadable to unauthorized users. Regularly updating software:- Keeping all systems and software updated ensures that known vulnerabilities are patched.
- Using multi-factor authentication (MFA): MFA adds an additional layer of security by requiring multiple forms of verification before granting access.
- Conducting regular security audits: Regularly reviewing and assessing the organization's security posture can help identify potential vulnerabilities.
- Educating employees: Training staff about the importance of data security and best practices can reduce the risk of human errors leading to data breaches.
-
Why is data growth a challenge?
Data growth poses a challenge because as data volumes increase, it becomes more difficult to manage, store, and process the information efficiently. Larger datasets can slow down systems, require more storage capacity, and increase costs. Additionally, with more data comes the challenge of ensuring its accuracy, relevance, and security. Organizations need to invest in scalable infrastructure and advanced data management tools to handle the surge in data effectively. -
What is data transformation?
Data transformation refers to the process of converting data from one format, structure, or type to another. This process is essential for various tasks such as data integration, data migration, and data warehousing. It involves cleaning, enriching, and reformatting the data to make it more suitable for a specific purpose, such as analysis or reporting.